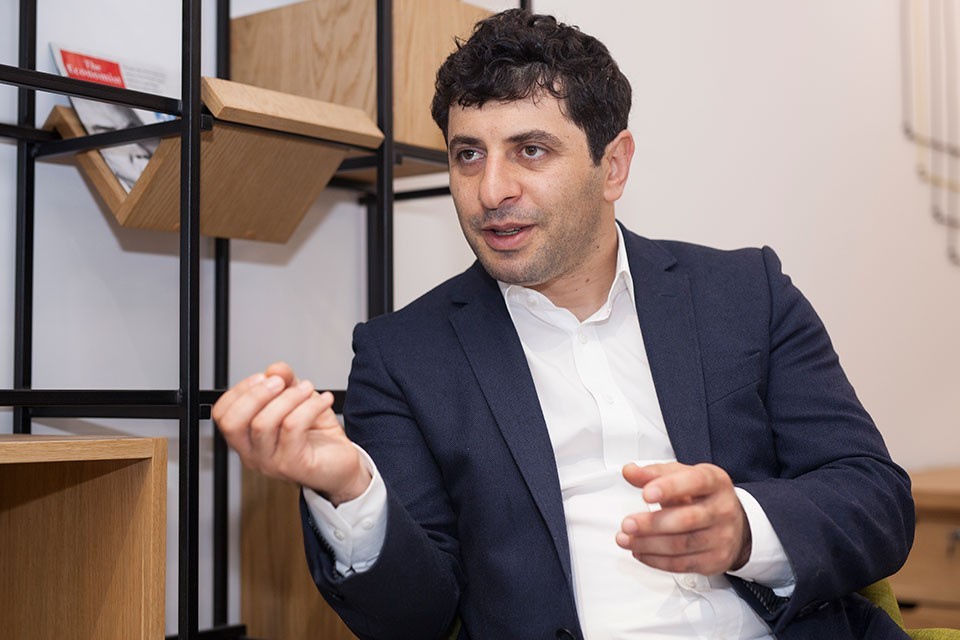
Blending Human Intelligence and Machine Learning to Make Investment Decisions
Blending Human Intelligence and Machine Learning to Make Investment Decisions
In these turbulent times, everyone is struggling to make sense of the geopolitical risks that are on the horizon, whether it be the finance industry making daily investments decisions, or individuals deciding who they will vote for in their next election. Gagik Sargsyan is the CEO of Polixis, a firm that uses technology to classify and assess regulatory, sanction and Anti-Money Laundering (AML) risks for investment decision-making. We sat down with Gagik to better understand how data and technology can help and where the limitations of machines lay.
*Note: this interview has been cut for breadth and clarity.
First, tell us about you and your company.
After finishing my MA in International Affairs at the Graduate Institute (IHEID), I was offered a job at HSBC in political and corruption risk analysis. Life is unpredictable. At the time, I never would’ve thought I would end up in banking, but I saw it as quite serendipitous and took the role. Subsequently, I moved to BNP Paribas for few years doing the same type of work.
At a certain point, I became bored and decided to take a leap of faith to create my own company. This was more or less a decade ago. After a number of successful projects advising clients on corruption, political and money laundering risks, we decided to become a technological company. As a risk professional we were using the existing tools sets at the time and were not quite satisfied with them. We observed that the market was lacking full circle, interconnected compliance data. We decided to fully immerse ourselves and became the first Swiss company in the field, and a leading global player when it comes to emerging markets.
Today we employ around 80 employees in Europe and Asia. We have offices in Zurich, London and Yerevan and we are constantly recruiting new collaborators. So, if you are a social scientist or a programmer, you can most certainly find a job with us! In fact, we employ more than a dozen social scientist to conduct detailed research to constantly update our algorithm with the latest developments and ensure a high standard of the data sets we use to train our AI.
Why are Anti-Money Laundering systems important to investors? How do they relate to ESG frameworks?
In a sense, it is already a core component of any ESG investment decision because running corruption risk analysis is linked to social and governmental risks. Ultimately, AML diligence has been the bedrock of financial system for at least 20 years. After the traumatic events of 9/11, terrorist financing, anti-money laundering and corruption risks have gained importance in the banking community globally. In Switzerland, it is no longer a choice but an obligation to ensure that such type of due diligence is duly and systematically carried out.
However, like ESG criteria, the issue is that money laundering and corruption risks tend to also include broader political risks. As we all know, political risks include a vast array of considerations and often depend on the definition of risk, as well as the entity you are conducting the analysis for. Nevertheless, the largest chunk of corruption risk analysis we undertake is to identify and evaluate what we call politically exposed persons (PEP) and politically exposed companies (PEC). These are people holding senior political positions who are being entrusted with prominent public functions and, therefore, may have a larger corruption risk than others. Our analysis contributes to building a better understanding of the environment in which the investor evolves and may help to identify and mitigate other ESG-related risks as well.
We try to distinguish whether a connection with these people constitutes a corruption risk or may bring about some sort of reputational risk for the investor. The challenge is not so much in screening out clear cases of corrupt officials, but rather in trying to evaluate the cases that are in the grey areas where different opposing opinions are held. As for human right violation cases, classifying infringement or not is an easier exercise with current AI technology, however, the more difficult philosophical question is determining what is an infringement. Having access to structured data sets and analysis enables better understanding of the context and underscores certain patterns that would have been harder to shed a light on based solely on manual analysis.
Why not enter directly the ESG scoring market?
Well, the big challenge in the ESG field is having proper frameworks and definitions that can be translated from a technological standpoint into classification and analysis with AI. The real question is classifying against what? Of course, one could simply follow PRI or the latest UN guidance, however, everybody in the industry understands how hard decision-making is in practice. Furthermore, risk definitions are very often in the eye of the beholder, which makes them more context-dependent and therefore harder to implement following a very general framework.
In that sense, there are similarities between the corruption risk analysis and ESG scoring field. For instance, even in a straightforward situation of classifying a politically exposed company, there still exists a large grey zone. Often, the issues do not lie in the technology but rather in the philosophical definition underpinning the classification. Hence, challenging issues require us first to revisit the definitions used and where possible, improve them. Then, the technological solutions are rather straightforward. However, you also need to ensure that the market agrees with you. This is quite a hurdle as you must convince each and every player that you took the right approach, and that is quite a time-consuming task.
What are the current technological challenges in your field? Are there limitations on what Artificial Intelligence and big data can do?
The biggest problem of the industry is not necessarily developing or processing AI models. Year over year, AI becomes easier to implement and scale for more complex problems. However, the core challenge is having proper data sets to train the algorithms on.
For us, data treatment and processing are key comparative advantages, and we are one of the leaders in that field. We pour a lot of effort into ensuring high data standards and try to refine them as much as possible to ensure that the data we train our algorithm on are well structured. We constantly try to improve the existing technological infrastructure, however, at the same time, we acknowledge the limitations of our technology and the fact that it cannot answer all our problems. Sometimes, answering a question simply requires common-sense decisions that only human being can make. Although you cannot completely automate everything, AI and big data enable identification of connections that would be harder to see otherwise.
In your view, how important are money-laundering and corruption risk barriers to scaling-up sustainable investments, especially in emerging markets?
Very often when you invest in emerging market or markets that are opaque and which have a lack of data or a lack of civil society control over public decision making processes, etc. there are important questions that need to be answered early on, and an extensive due diligence process is key. These questions might include whether the project is linked with politically exposed persons or members of their family. For instance, is it an ESG risk to be dealing with a very politically powerful person in a fragile society where this person can bend the laws and make sure that the project looks perfect? Lacking solid answers may disincentivise investors to enter in a field they do not sufficiently understand, hence, creating a barrier and reinforcing at-home biases.
You may not need technology to find out who these people are. However, it can be very interesting to see who is connected to whom and on what basis. Manually, you may never think to check so much data or you would only do so if you already know what you are looking for. Leaving this to a machine to pre-process enables a better understanding of the overall environment and helps to save time in the acquisition of key insights on the interactions between different actors.
Nonetheless, ultimately, you need to have good and competent consultants that are specialized in a certain market to fully overcome this local barrier. AI and big data can substantially help, but there is always a limit to what a machine can do.